Man can teach computers to do many things better than humans can today; in fact, computers can teach other computers to do so. In recent years, spectacular progress has been made in the world of artificial intelligence (AI) and “deep learning” (machine-learning methods based on artificial neural networks that have been applied to fields including computer vision, speech recognition, natural language processing, machine translation, bioinformatics, medical image analysis, material inspection and board game programs, where they have produced results comparable to and in some cases surpassing human expert performance).
Despite their great promise, AI systems have yet to become ubiquitous in the daily practice of medicine largely due to several crucial unmet needs of healthcare practitioners. These include lack of explanations in clinically meaningful terms, handling the presence of unknown medical conditions and transparency regarding the system’s limitations, both in terms of statistical performance as well as recognizing situations for which the system’s predictions are irrelevant.
So at present, there are virtually no medical products on the shelf that use this technology, and as a result, doctors continue to use the same tools used in previous decades.
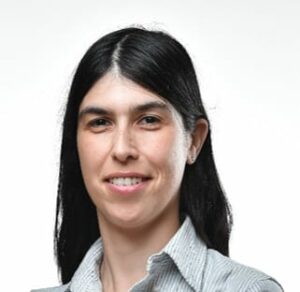
To find a solution to this problem, the research group of Prof. Yael Yaniv of the Faculty of Biomedical Engineering at the Technion-Israel Institute of Technology in Haifa joined forces with the research groups of Prof. Alex Bronstein and Prof. Assaf Schuster of the Taub Faculty of Computer Science to create an AI-based system that automatically detects disease on the basis of hundreds of electrocardiograms, which are currently the most widespread technology employed for the diagnosis of cardiac pathology.
Elul and Aviv Rosenberg Now, under their joint supervision, research by doctoral students Yonatan has been published in Proceedings of the National Academy of Sciences of the United States of America (PNAS) under the title “Meeting the unmet needs of clinicians from AI systems showcased for cardiology with deep-learning–based ECG analysis.
The new system automatically analyzes the electrocardiograms (ECGs) using augmented neural networks – the most prominent tool in deep learning today. These networks learn different patterns by training on a large number of samples, and the system developed by the researchers was trained on more than 1.5 million ECG segments sampled from hundreds of patients in hospitals in various countries.
The electrocardiogram, developed more than 100 years ago, provides important information on conditions affecting the heart quickly and non-invasively. The problem is that the printouts have to be interpreted by a human cardiologist, so their interpretation is – by necessity – pervaded by subjective elements. As a result, numerous research groups around the world are working to develop systems that will automatically interpret the printouts efficiently and accurately. These systems are also able to identify pathological conditions that human cardiologists –
regardless of their experience – are unable to detect.
The Technion system was built according to the needs set down by cardiologists, and its output includes an uncertainty estimation of the results, indication of suspicious areas on the ECG wave and alerts regarding inconclusive results and increased risk of pathology not observed in the ECG signal itself.
The system is sensitive enough to provide alerts regarding patients at risk of arrhythmia (irregular heartbeat) – even when the arrhythmia is not shown on the ECG printout. In addition, the rate of false alarms is very minimal. The new system also explains its decisions using the accepted cardiology terminology.
The researchers hope their system can be used for cross-population scanning for the early detection of those who are at risk of arrhythmia. Without this early diagnosis, these people have an increased risk of heart attack and stroke.
The shortcode is missing a valid Donation Form ID attribute.